|
ACCESS THE FULL ARTICLE
No SPIE Account? Create one
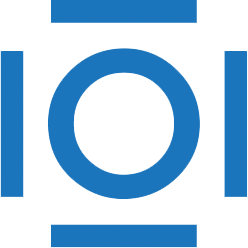
CITATIONS
Cited by 1 scholarly publication.
Personal digital assistants
Sensors
Detection and tracking algorithms
Filtering (signal processing)
Probability theory
Kinematics
Data modeling