|
ACCESS THE FULL ARTICLE
No SPIE Account? Create one
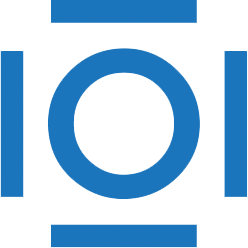
CITATIONS
Cited by 4 scholarly publications.
Sensors
Target recognition
Iron
Signal to noise ratio
Image compression
Image sensors
Target detection