|
ACCESS THE FULL ARTICLE
No SPIE Account? Create one
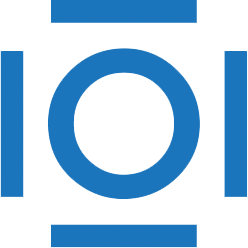
CITATIONS
Cited by 2 scholarly publications.
Speech recognition
Signal to noise ratio
Electronic filtering
Gaussian filters
Linear filtering
Interference (communication)
Neural networks