|
ACCESS THE FULL ARTICLE
No SPIE Account? Create one
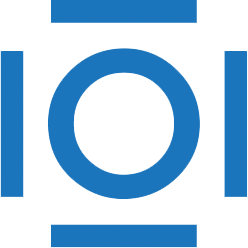
CITATIONS
Cited by 1 scholarly publication.
Neural networks
Wavelets
Image classification
Wavelet transforms
Linear filtering
Statistical analysis
Evolutionary algorithms