|
ACCESS THE FULL ARTICLE
No SPIE Account? Create one
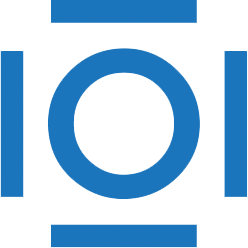
CITATIONS
Cited by 9 scholarly publications.
Data modeling
Filtering (signal processing)
Modeling
Neural networks
Stochastic processes
Detection and tracking algorithms
Linear filtering