|
ACCESS THE FULL ARTICLE
No SPIE Account? Create one
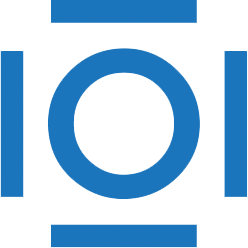
CITATIONS
Cited by 1 scholarly publication.
Calibration
Neural networks
Data modeling
System identification
Detection and tracking algorithms
Factor analysis
Statistical analysis