|
ACCESS THE FULL ARTICLE
No SPIE Account? Create one
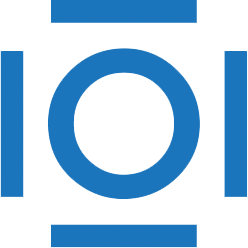
CITATIONS
Cited by 4 scholarly publications.
Wavelets
Statistical analysis
Heart
Fractal analysis
Wavelet transforms
Signal processing
Data modeling