|
ACCESS THE FULL ARTICLE
No SPIE Account? Create one
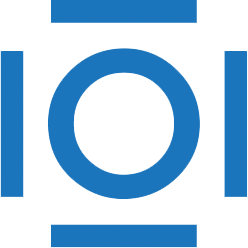
CITATIONS
Cited by 3 scholarly publications.
Stars
Data modeling
Probability theory
Sensors
Error analysis
Charge-coupled devices
Interference (communication)