|
ACCESS THE FULL ARTICLE
No SPIE Account? Create one
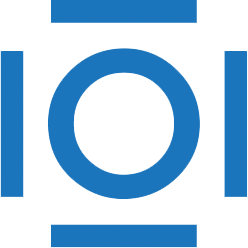
CITATIONS
Cited by 1 scholarly publication.
Data modeling
System identification
Artificial neural networks
Binary data
Signal generators
Evolutionary algorithms
Computer engineering