|
ACCESS THE FULL ARTICLE
No SPIE Account? Create one
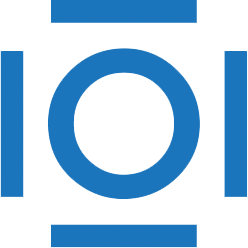
CITATIONS
Cited by 5 scholarly publications.
Neural networks
Optical character recognition
Artificial neural networks
Classification systems
Binary data
Image classification
Computer engineering