|
ACCESS THE FULL ARTICLE
No SPIE Account? Create one
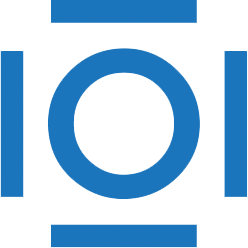
CITATIONS
Cited by 8 scholarly publications.
Neural networks
Performance modeling
Artificial intelligence
Machine vision
Robotics
Visual process modeling
Visualization