|
ACCESS THE FULL ARTICLE
No SPIE Account? Create one
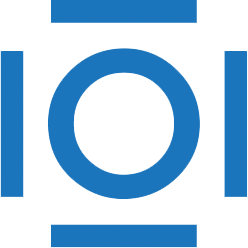
CITATIONS
Cited by 1 scholarly publication.
Hyperspectral imaging
Visualization
Image classification
3D visualizations
Image visualization
Pattern recognition
Remote sensing