|
ACCESS THE FULL ARTICLE
No SPIE Account? Create one
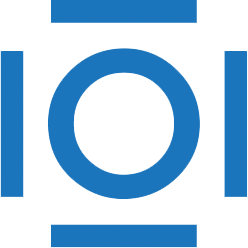
CITATIONS
Cited by 1 scholarly publication.
Gait analysis
Parkinson's disease
Kinematics
Statistical analysis