|
ACCESS THE FULL ARTICLE
No SPIE Account? Create one
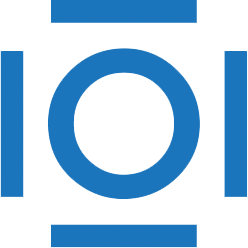
CITATIONS
Cited by 1 scholarly publication.
Image segmentation
Retina
Optical coherence tomography
Data modeling
Convolutional neural networks
Eye
Animal model studies