|
ACCESS THE FULL ARTICLE
No SPIE Account? Create one
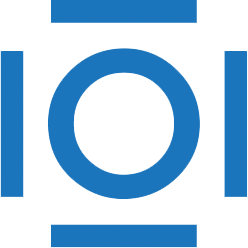
CITATIONS
Cited by 1 scholarly publication.
Image registration
Magnetic resonance imaging
Computed tomography
Radiotherapy
Rectum
3D modeling
Cancer