|
ACCESS THE FULL ARTICLE
No SPIE Account? Create one
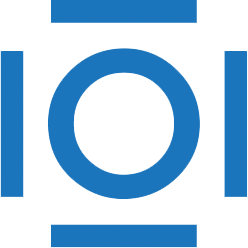
CITATIONS
Cited by 2 scholarly publications.
Magnetic resonance imaging
Scanning probe microscopy
Data acquisition
Image filtering
Data modeling
Neural networks
Autoregressive models