|
ACCESS THE FULL ARTICLE
No SPIE Account? Create one
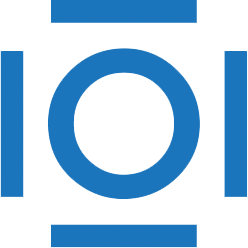
CITATIONS
Cited by 3 scholarly publications.
Detection and tracking algorithms
Diffusion
Mathematical modeling
Social networks
Algorithm development
Inverse problems