|
ACCESS THE FULL ARTICLE
No SPIE Account? Create one
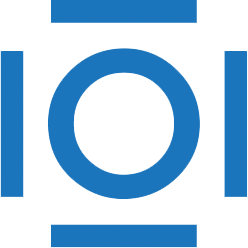
CITATIONS
Cited by 1 scholarly publication.
Positron emission tomography
Image restoration
Computed tomography
Denoising
Neural networks
Image quality
Reconstruction algorithms