|
ACCESS THE FULL ARTICLE
No SPIE Account? Create one
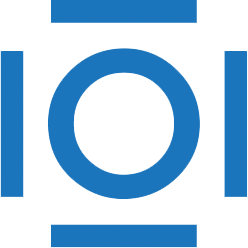
CITATIONS
Cited by 4 scholarly publications.
Sensors
Infrared sensors
Neural networks
Image classification
Classification systems
Feature extraction
Detection and tracking algorithms