|
ACCESS THE FULL ARTICLE
No SPIE Account? Create one
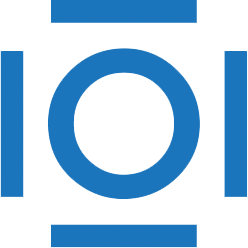
CITATIONS
Cited by 2 scholarly publications.
Video surveillance
Optical tracking
Feature extraction
Network architectures
Detection and tracking algorithms