|
ACCESS THE FULL ARTICLE
No SPIE Account? Create one
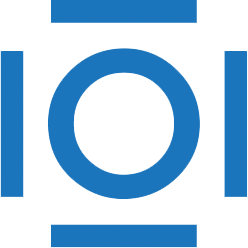
CITATIONS
Cited by 1 scholarly publication.
Denoising
Data modeling
Neural networks
Acoustics
Acoustic emission
Feature extraction
Sensors