|
ACCESS THE FULL ARTICLE
No SPIE Account? Create one
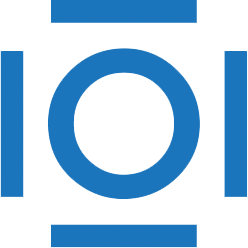
CITATIONS
Cited by 2 scholarly publications.
Fuzzy logic
Data centers
Algorithms
Machine learning
Optimization (mathematics)
Visualization
Fluctuations and noise