|
ACCESS THE FULL ARTICLE
No SPIE Account? Create one
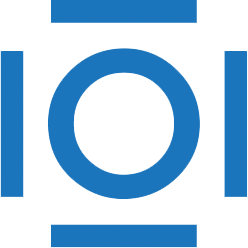
CITATIONS
Cited by 3 scholarly publications and 1 patent.
Endoscopy
Feature extraction
Neurons
Convolution
Lithium
Data modeling
Machine learning