|
ACCESS THE FULL ARTICLE
No SPIE Account? Create one
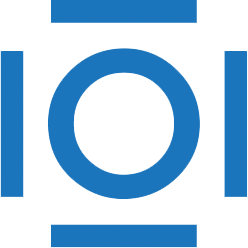
CITATIONS
Cited by 4 scholarly publications.
Tissues
Image segmentation
Image filtering
Machine learning
Wound healing
Feature extraction
Mobile devices