|
ACCESS THE FULL ARTICLE
No SPIE Account? Create one
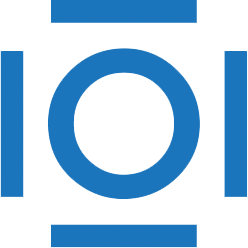
CITATIONS
Cited by 2 scholarly publications.
Feature extraction
Principal component analysis
Kinematics
Independent component analysis
Robotics
Surgery
Computer simulations