|
ACCESS THE FULL ARTICLE
No SPIE Account? Create one
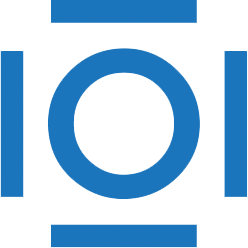
CITATIONS
Cited by 2 scholarly publications and 2 patents.
Convolutional neural networks
3D modeling
Data modeling
Fractal analysis
Volume rendering
Error analysis
Image analysis