|
ACCESS THE FULL ARTICLE
No SPIE Account? Create one
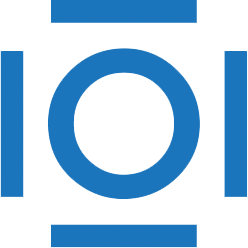
CITATIONS
Cited by 13 scholarly publications and 1 patent.
Algorithm development
Unmanned aerial vehicles
Computer vision technology
Machine vision
Neural networks
Agriculture
Convolution