|
ACCESS THE FULL ARTICLE
No SPIE Account? Create one
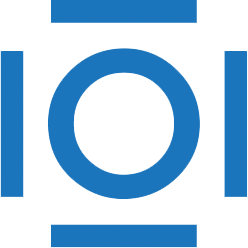
CITATIONS
Cited by 35 scholarly publications.
Satellite imaging
Satellites
Earth observing sensors
Feature extraction
Principal component analysis
Convolutional neural networks
Magnetorheological finishing