|
ACCESS THE FULL ARTICLE
No SPIE Account? Create one
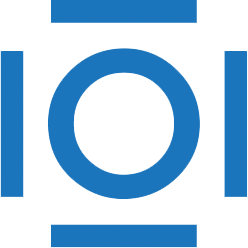
CITATIONS
Cited by 1 scholarly publication.
Databases
Facial recognition systems
Detection and tracking algorithms
Computer programming
Error control coding
Principal component analysis
Optical spheres