|
ACCESS THE FULL ARTICLE
No SPIE Account? Create one
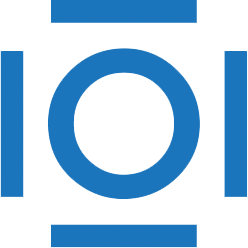
CITATIONS
Cited by 1 scholarly publication.
Expectation maximization algorithms
Data modeling
Visual process modeling
Statistical modeling
Optimization (mathematics)
Statistical analysis
Computer vision technology