|
ACCESS THE FULL ARTICLE
No SPIE Account? Create one
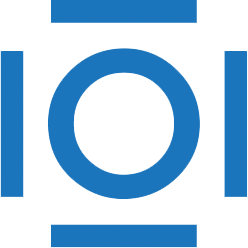
CITATIONS
Cited by 2 scholarly publications.
Feature selection
Feature extraction
Classification systems
Detection and tracking algorithms
Data modeling
Databases
Sensors