|
ACCESS THE FULL ARTICLE
No SPIE Account? Create one
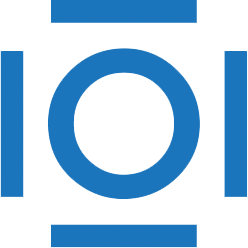
CITATIONS
Cited by 2 scholarly publications.
Data modeling
Visualization
Statistical analysis
Error analysis
Fuzzy logic
Genetic algorithms
Statistical modeling