|
ACCESS THE FULL ARTICLE
No SPIE Account? Create one
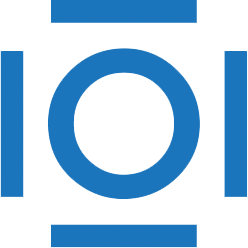
CITATIONS
Cited by 2 scholarly publications.
Diffusion
Anisotropic diffusion
Smoothing
Edge detection
Image processing
Nonlinear filtering
Gaussian filters