|
ACCESS THE FULL ARTICLE
No SPIE Account? Create one
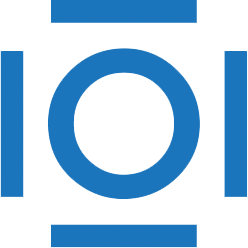
CITATIONS
Cited by 2 scholarly publications.
Image processing
Magnetorheological finishing
Image restoration
Binary data
Image analysis
Data modeling
Stochastic processes