|
ACCESS THE FULL ARTICLE
No SPIE Account? Create one
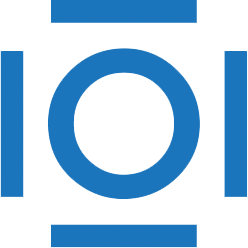
CITATIONS
Cited by 2 scholarly publications.
Error analysis
Statistical modeling
Aerospace engineering
Data modeling
Neural networks
Neurons
Detection and tracking algorithms