|
ACCESS THE FULL ARTICLE
No SPIE Account? Create one
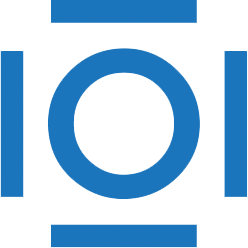
CITATIONS
Cited by 1 scholarly publication.
Tumors
Lung cancer
Image segmentation
Data modeling
Computed tomography
Feature extraction
Tumor growth modeling