|
ACCESS THE FULL ARTICLE
No SPIE Account? Create one
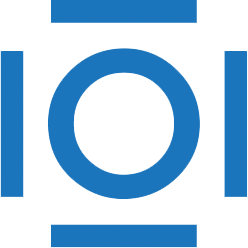
CITATIONS
Cited by 1 scholarly publication.
Video
Convolution
Quantization
3D modeling
Machine vision
Computer vision technology