|
ACCESS THE FULL ARTICLE
No SPIE Account? Create one
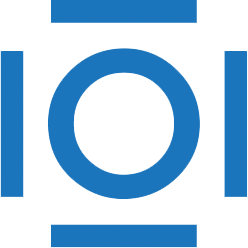
CITATIONS
Cited by 1 scholarly publication.
Feature extraction
Principal component analysis
Optical spheres
Computer programming
Computer programming languages
Convolutional neural networks
Image classification