|
ACCESS THE FULL ARTICLE
No SPIE Account? Create one
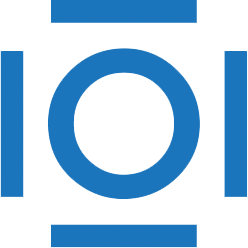
CITATIONS
Cited by 1 scholarly publication.
3D modeling
Data modeling
3D image processing
3D image reconstruction
Performance modeling
Unmanned aerial vehicles
3D scanning