|
ACCESS THE FULL ARTICLE
No SPIE Account? Create one
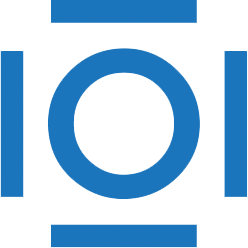
CITATIONS
Cited by 1 scholarly publication.
Breast
Image segmentation
Convolutional neural networks
Image processing
Mammography
Computing systems
Machine learning