|
ACCESS THE FULL ARTICLE
No SPIE Account? Create one
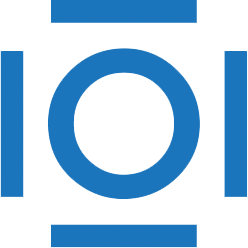
CITATIONS
Cited by 3 scholarly publications.
Particle swarm optimization
Object recognition
Particles
Detection and tracking algorithms
Scanning probe microscopy
Feature extraction
Databases