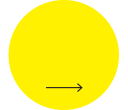
Search the world's largest collection of optics and photonics applied research.
Announcements
REVIEWER TRAINING PROGRAM
Become a great peer reviewer! This essential peer review training course offers a blend of online learning and hands-on peer review experience.
Get Started with the Reviewer Training Program
ENGLISH LANGUAGE EDITING SERVICES
SPIE partners with Editage to offer English language editing services to researchers worldwide.
15% discount applied at checkout
TECHNOLOGIES
The most viewed articles by technology, from SPIE Journals and Proceedings
Origins Space Telescope: baseline mission concept
J. Astronomical Telescopes, Instruments, and Systems (2021)
The Copernicus CO2M mission for monitoring anthropogenic carbon dioxide emissions from space
Proceedings of SPIE (2021)
Identification of different types of tumors based on photoacoustic spectral analysis: preclinical feasibility studies on skin tumors
J. of Biomedical Optics (2023)
Kernel Flux: a whole-head 432-magnetometer optically-pumped magnetoencephalography (OP-MEG) system for brain activity imaging during natural human experiences
Proceedings of SPIE (2021)
High capacity and access rate, data storage using laser communications
Optical Engineering (2021)
Modular FSO Optical System Design for Classical and Quantum Optical Communication Systems
Proceedings of SPIE (2021)
Survey on deep learning applications in digital image security
Optical Engineering (2021)
Tactical agility for AI-enabled multi-domain operations
Proceedings of SPIE (2023)
Efficient detection of crossing pedestrians from a moving vehicle with an array of cameras
Optical Engineering (2022)
Research on bridge defect detection technology based on image processing technology
Proceedings of SPIE (2023)
Holographic low concentration optical system increasing light collection efficiency of regular solar panels
J. Photonics for Energy (2021)
GALACTIC: high performance alexandrite crystals and coatings for high power space applications
Proceedings of SPIE (2021)
Structured light for ultrafast laser micro- and nanoprocessing
Optical Engineering (2021)
Development of LISA laser system at NASA
Proceedings of SPIE (2023)
Speckle control for electro-holographic display using high-brightness yellow phosphor light source in projector
Optical Engineering (2023)
Modular FSO Optical System Design for Classical and Quantum Optical Communication Systems
Proceedings of SPIE (2021)
Potential of E-beam lithography for micro- and nano-optics fabrication on large areas
J. Micro/Nanolithography, MEMS, and MOEMS (2023)
High-NA EUV Lithography exposure tool: advantages and program progress
Proceedings of SPIE (2021)
Enabling precision coordinate metrology for universal optical testing and alignment applications
Optical Engineering (2021)
Novel diffraction-based overlay metrology utilizing phase-based overlay for improved robustness
Proceedings of SPIE (2021)
Graphene/molybdenum disulfide nanocomposites: characterization and optoelectronic application
J. Nanophotonics (2023)
Carbon nanotube EUV pellicle tunability and lifetime
Proceedings of SPIE (2021)
Opto-thermo-mechanical phenomena in satellite free-space optical communications: survey and challenges
Optical Engineering (2023)
Optical communication requirements for scientific missions and the Deep Space Gateway
Proceedings of SPIE (2023)
Satellite remote sensing of river discharge: a framework for assessing the accuracy of discharge estimates made from satellite remote sensing observations
J. Applied Remote Sensing (2023)
A synchronized remote sensing monitoring approach in the Livingstone island region of Antarctica
Proceedings of SPIE (2023)
Technical concepts of automotive LiDAR sensors: a review
Optical Engineering (2023)
New developments of multilinear and multispectral infrared sensors for space applications at LYNRED
Proceedings of SPIE (2023)