|
ACCESS THE FULL ARTICLE
No SPIE Account? Create one
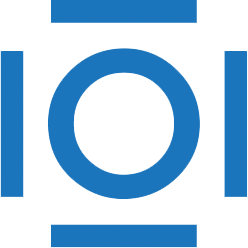
CITATIONS
Cited by 34 scholarly publications and 1 patent.
Neural networks
Image compression
Image quality
Statistical analysis
Feature selection
Principal component analysis
Data modeling