|
ACCESS THE FULL ARTICLE
No SPIE Account? Create one
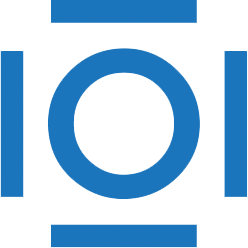
CITATIONS
Cited by 1 scholarly publication.
Ridge detection
Interference (communication)
Digital imaging
Image analysis
Medical imaging
Sensors
Statistical analysis