|
ACCESS THE FULL ARTICLE
No SPIE Account? Create one
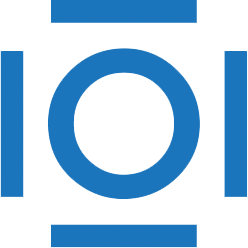
CITATIONS
Cited by 14 scholarly publications and 1 patent.
Calibration
Neural networks
Neurons
Associative arrays
Sensors
Geometrical optics
Distortion